2. Setting up Your Python Environment#
2.1. Overview#
In this lecture, you will learn how to
get a Python environment up and running
execute simple Python commands
run a sample program
install the code libraries that underpin these lectures
use Python in the cloud
2.2. Local Install#
Local installs are preferable if you have access to a suitable machine and plan to do a substantial amount of Python programming.
Local installs require sufficient memory and good processor capacity, as well as more work for configuration.
The rest of this lecture, except the last section, runs you through the some details associated with local installs.
2.3. Integrated Development Environment (IDE) for Python#
An integrated development environment (IDE) refers to a software application that offers computer programmers with extensive software development abilities. IDEs most often consist of a source code editor, build automation tools, and a debugger. Most modern IDEs have intelligent code completion.
In short terms:
An IDE enables programmers to combine the different aspects of writing a computer program.
IDEs increase programmer productivity by introducing features like editing source code, building executables, and debugging.
IDEs and Code Editors:
IDEs and code editors are tools that software developers use to write and edit code.
IDEs, or Integrated Development Environments, are usually more feature-rich and include tools for debugging, building and deploying code.
Code editors are generally more straightforward and focused on code editing. Many developers use IDEs and code editors, depending on the task.
IDE vs. Code Editor: What’s the Difference?
An Integrated Development Environment (IDE) is a software application that provides tools and resources to help developers write and debug code. An IDE typically includes
A source code editor
A compiler or interpreter
An integrated debugger
A graphical user interface (GUI)
A code editor is a text editor program designed specifically for editing source code. It typically includes features that help in code development, such as syntax highlighting, code completion, and debugging.
The main difference between an IDE and a code editor is that an IDE has a graphical user interface (GUI) while a code editor does not.
An IDE also has features such as code completion, syntax highlighting, and debugging, which are not found in a code editor.
Code editors are generally simpler than IDEs, as they do not include many other IDE components. As such, code editors are typically used by experienced developers who prefer to configure their development environment manually.
Some of the most used IDE for Python are:
IDLE
Spyder
Jupyter
Visual Studio Code
PyCharm
2.4. IDLE#
IDLE (Integrated Development and Learning Environment) is a default editor that accompanies Python, so is free. This IDE is suitable for beginner-level developers and can be used on Mac OS, Windows, and Linux.
The most notable features of IDLE include:
Ability to search for multiple files.
Interactive interpreter with syntax highlighting, and error and i/o messages.
Smart indenting, along with basic text editor features.
A very capable debugger.
Its a great Python IDE for Windows.
An example of the IDLE window is:
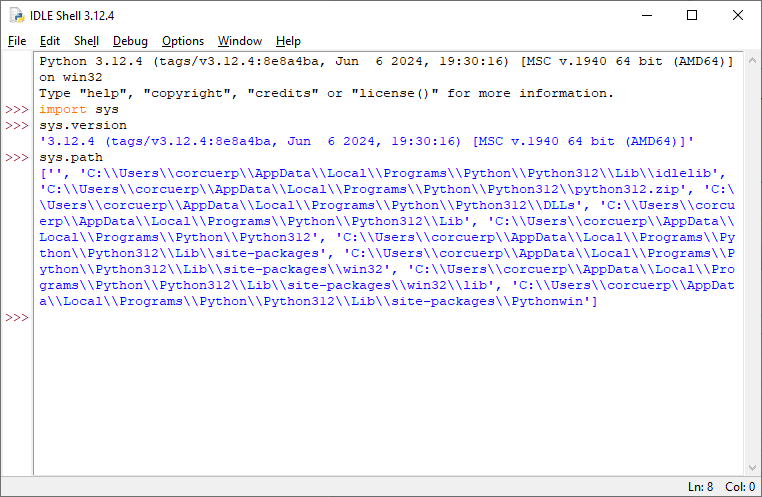
2.5. Spyder#
Spyder is an open-source IDE most commonly used for scientific development. Spyder comes with Anaconda distribution, which is popular for data science and machine learning.
The most notable features of Spyder include:
Support for automatic code completion and splitting.
Supports plotting different types of charts and data manipulation.
Integration of data science libraries like NumPy, Pandas, and Matplotlib.
Its a great Python IDE for Windows.
For those who are used to Matlab, Spyder’s interface should immediately look familiar. Spyder’s standard look is shown in Fig. 2.1.
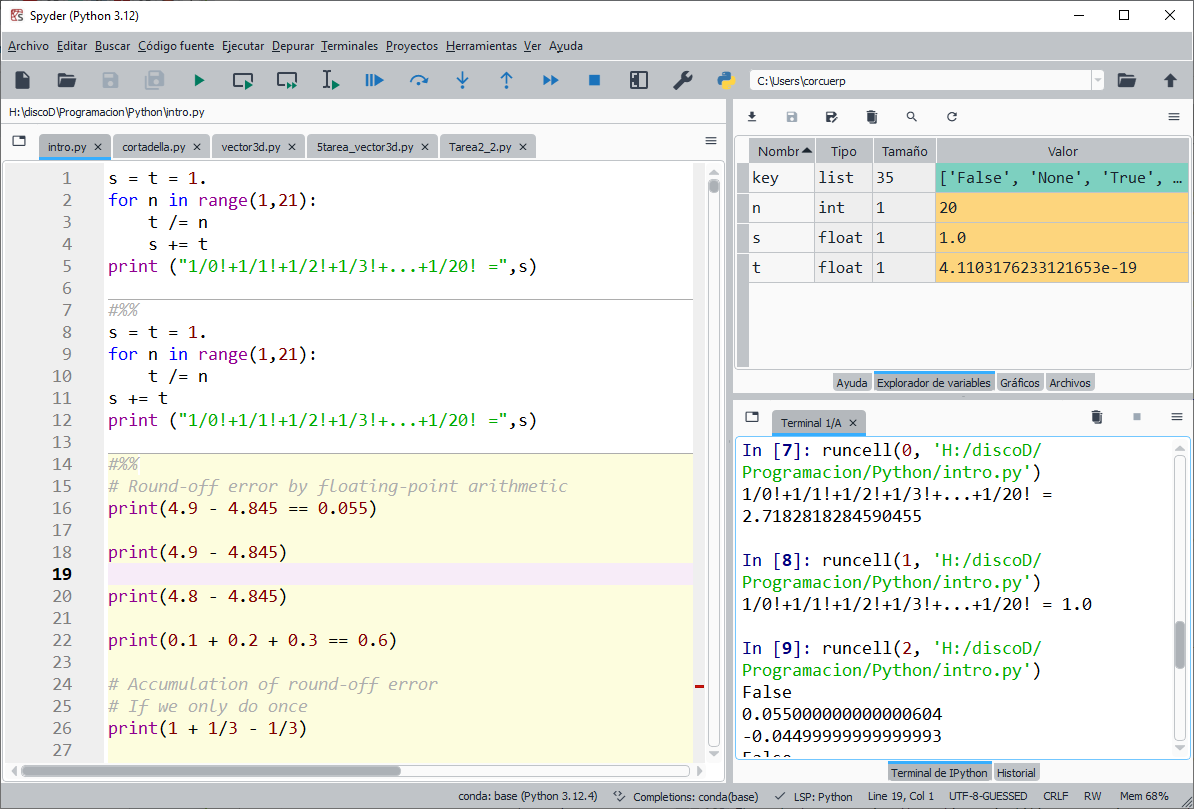
Fig. 2.1 The three main panes of the standard Spyder interface.#
2.5.1. Writing code in the console#
The right down pane of Fig. 2.1 is the console. It executes one command at a time. It also shows the result of calculations. Try entering this simple calculation in the console:
1 + 2
The console will show the results just below your command:
3
You can also write multiple-line commands using CTRL+Enter. Both are executed, but only the result from the last command is printed:
1 + 2
3 + 4
7
To print the result of every command, we explicitly use the print
command:
print("Hello world")
print("This is my first program")
Hello world
This is my first program
2.5.2. Writing code in a script#
The left section of Fig. 2.1 is the script editor. It is simply a text editor that allows saving code as a .py text file, to execute it all together later. You can execute a script by clicking on the “Run File” button (Fig. 2.2).
As an exercise, create a file that prints “Hello World”, save it as hello_world.py
, and run it using the
“Run File” button. You should see the text “Hello World” appear in the console.
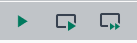
Fig. 2.2 “Run file”, “Run current cell”, “Run current cell and advance”.#
2.5.3. Code cell#
When a script grows in length, it can be practical to run only one section at a time. A script can be split into cells using this sequence of characters that acts as a separator:
# %%
You can also name cells by adding a title next to the separator:
# %% Load results from previous acquisition
[...]
# %% Report these results in a local coordinate system
[...]
You can execute a cell by placing the cursor in that cell, then by clicking on the “Run current cell” or “Run current cell and advance” button (Fig. 2.2).
As an exercise, create a file named exercise.py
that contains two cells. A first cell prints “Hello world”,
and a second cell prints “This is my first program”. Run the cell of your choice using the toolbar icons.
2.5.4. Getting help#
The right upper section C Fig. 2.1 is a collection of various panes.
The help pane is particularly helpful to navigate the documentation of a given module or package.
For example, to better understand how to use the Python max
function, write max
in the help pane.
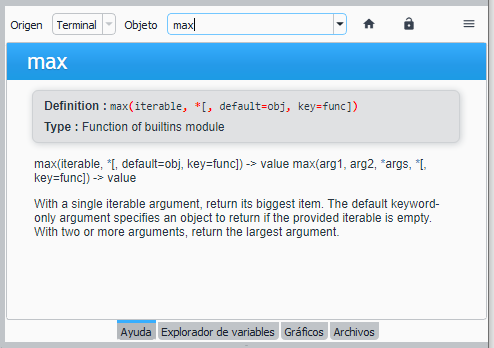
Fig. 2.3 Getting help on a function using Spyder’s help pane.#
Note that you can also get help from the console, using the help
function:
help(max)
Help on built-in function max in module builtins:
max(...)
max(iterable, *[, default=obj, key=func]) -> value
max(arg1, arg2, *args, *[, key=func]) -> value
With a single iterable argument, return its biggest item. The
default keyword-only argument specifies an object to return if
the provided iterable is empty.
With two or more arguments, return the largest argument.
2.6. Anaconda#
The core Python package is easy to install but not what you should choose for these lectures.
These lectures require the entire scientific programming ecosystem, which
the core installation doesn’t provide
is painful to install one piece at a time.
Hence the best approach for our purposes is to install a Python distribution that contains
the core Python language and
compatible versions of the most popular scientific libraries.
The best such distribution is Anaconda.
Anaconda is
very popular
cross-platform
comprehensive
Note
Anaconda also comes with a great package management system to organize your code libraries.
2.6.1. Installing Anaconda#
To install Anaconda, download the binary and follow the instructions.
Important points:
Install the latest version!
If you are asked during the installation process whether you’d like to make Anaconda your default Python installation, say yes.
2.6.2. Updating Anaconda#
Anaconda supplies a tool called conda
to manage and
upgrade your Anaconda packages.
One conda
command you should execute regularly is the one
that updates the whole Anaconda distribution.
As a practice run, please execute the following
Open up a terminal
Type
conda update anaconda
For more information on conda
, type conda help
in a terminal.
2.7. Jupyter Notebooks#
Jupyter notebooks are one of the many possible ways to interact with Python and the scientific libraries. Jupyter is widely used in the field of data science. It is easy to use, interactive and allows live code sharing and visualization.
The most notable features of Jupyter include:
Supports for the numerical calculations and machine learning workflow.
Combine code, text, and images for greater user experience.
Intergeneration of data science libraries like NumPy, Pandas, and Matplotlib.
Because of these features, Jupyter is now a major player in the scientific computing ecosystem.
They use a browser-based interface to Python with
The ability to write and execute Python commands.
Formatted output in the browser, including tables, figures, animation, etc.
The option to mix in formatted text and mathematical expressions.
Here’s an image showing execution of some code (borrowed from here) in a Jupyter notebook
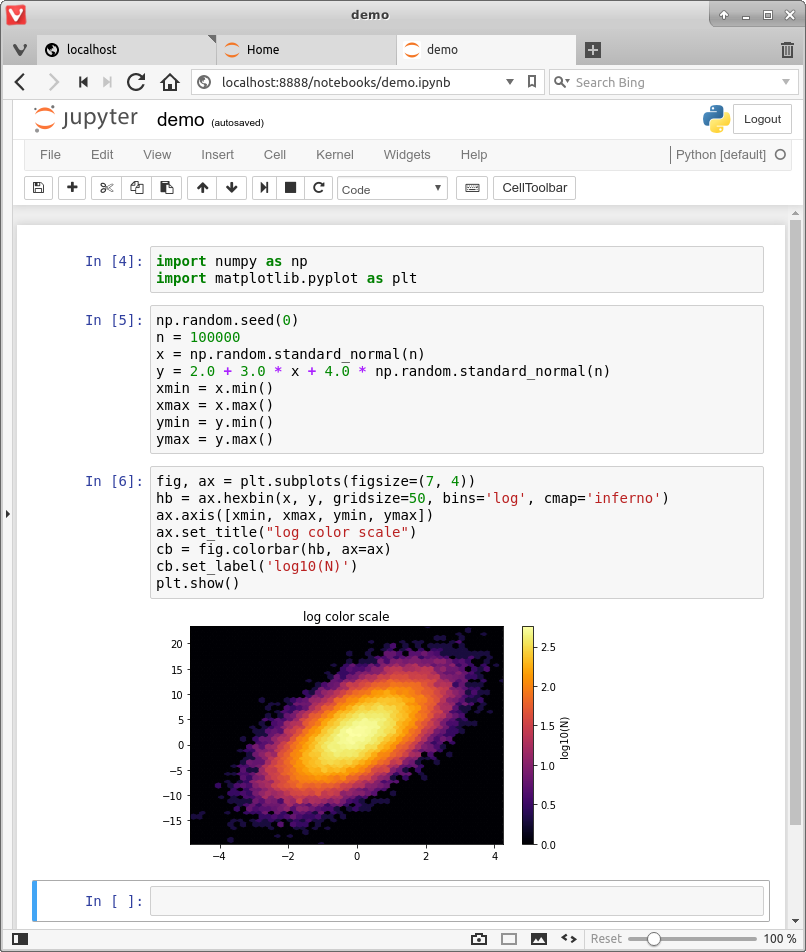
2.7.1. Starting the Jupyter Notebook#
Once you have installed Anaconda, you can start the Jupyter notebook.
Either
search for Jupyter in your applications menu, or
open up a terminal and type
jupyter notebook
Windows users should substitute “Anaconda command prompt” for “terminal” in the previous line.
If you use the second option, you will see something like this
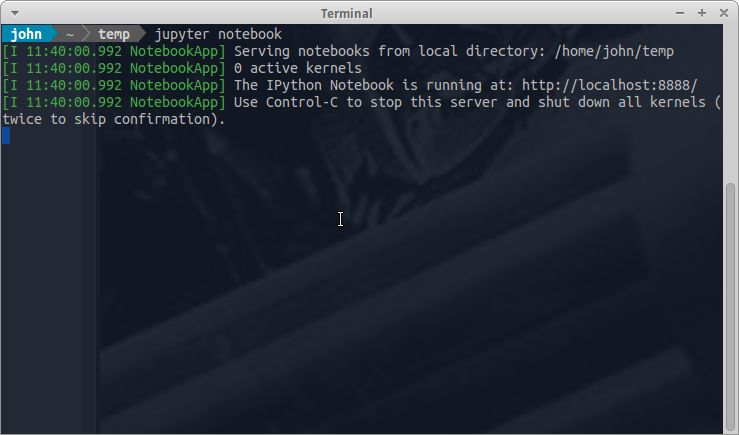
The output tells us the notebook is running at http://localhost:8888/
localhost
is the name of the local machine8888
refers to port number 8888 on your computer
Thus, the Jupyter kernel is listening for Python commands on port 8888 of our local machine.
Hopefully, your default browser has also opened up with a web page that looks something like this
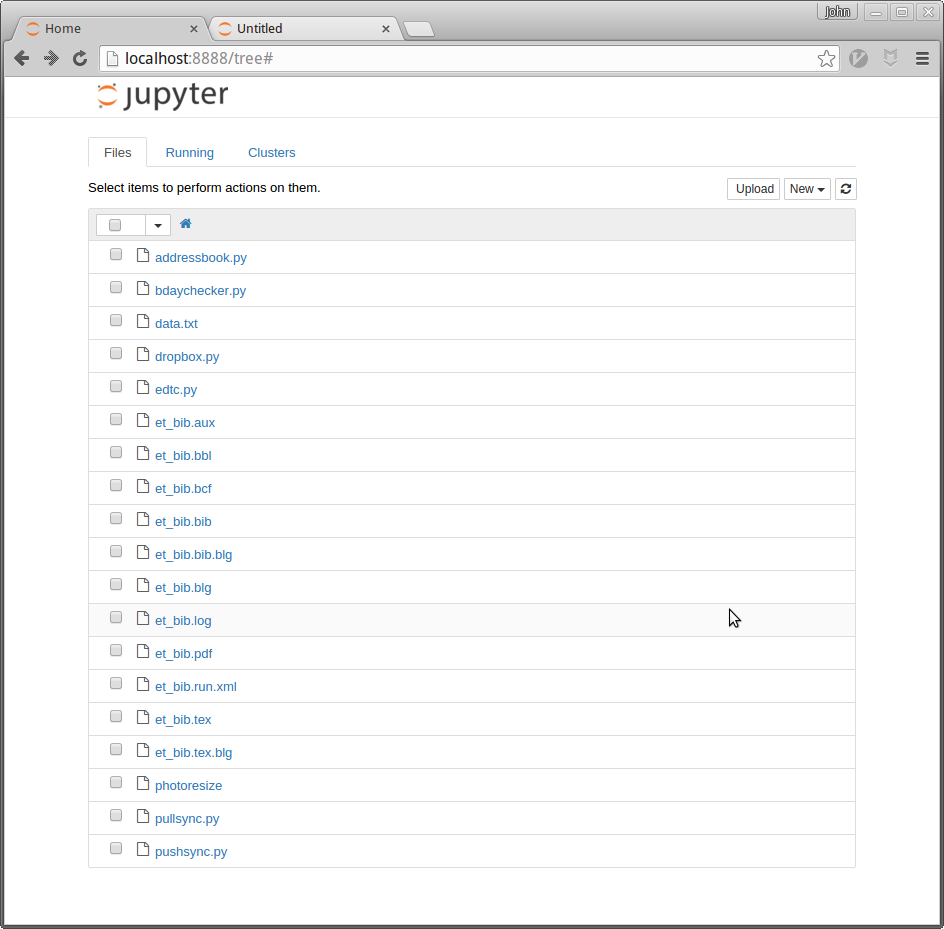
What you see here is called the Jupyter dashboard.
If you look at the URL at the top, it should be localhost:8888
or
similar, matching the message above.
Assuming all this has worked OK, you can now click on New
at the top
right and select Notebook
or similar.
Here’s what shows up on our machine:
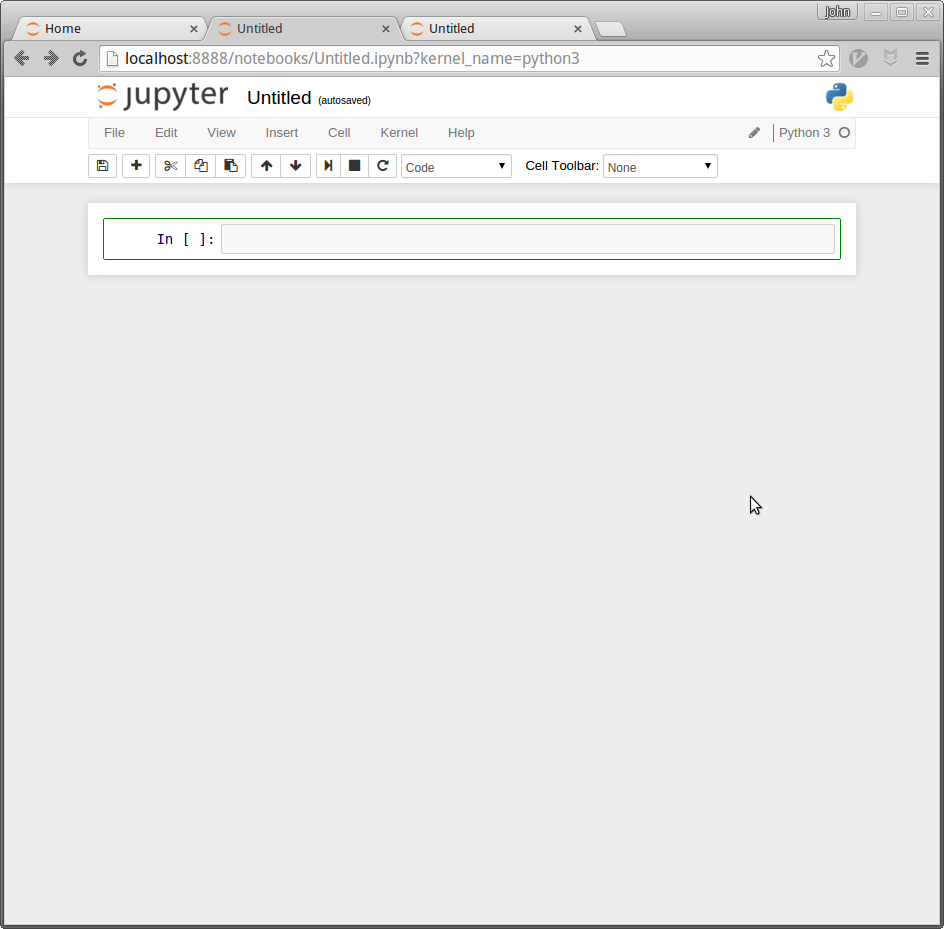
The notebook displays an active cell, into which you can type Python commands.
2.7.2. Notebook Basics#
Let’s start with how to edit code and run simple programs.
2.7.2.1. Running Cells#
Notice that, in the previous figure, the cell is surrounded by a blue border.
This means that the cell is in edit mode.
In this mode, whatever you type will appear in the cell with the flashing cursor.
When you’re ready to execute the code in a cell, hit Shift-Enter
instead of the usual Enter
.
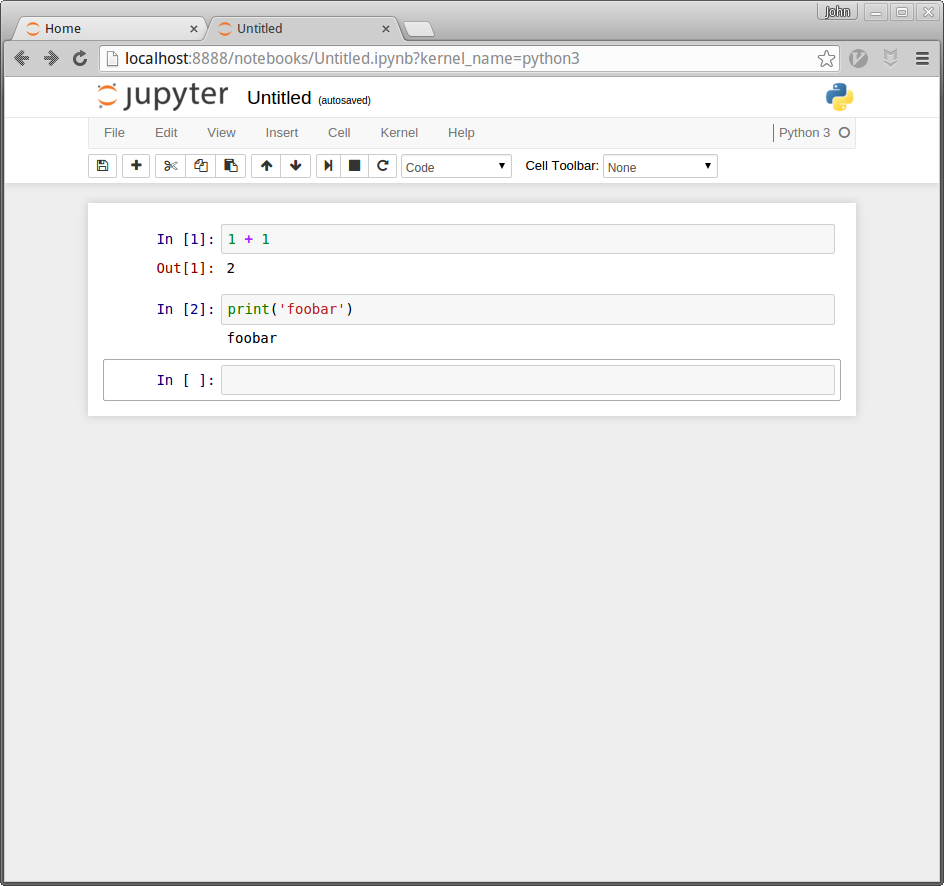
(Note: There are also menu and button options for running code in a cell that you can find by exploring)
2.7.2.2. Modal Editing#
The next thing to understand about the Jupyter notebook is that it uses a modal editing system.
This means that the effect of typing at the keyboard depends on which mode you are in.
The two modes are
Edit mode
Indicated by a green border around one cell, plus a blinking cursor
Whatever you type appears as is in that cell
Command mode
The green border is replaced by a grey (or grey and blue) border
Keystrokes are interpreted as commands — for example, typing
b
adds a new cell below the current one
To switch to
command mode from edit mode, hit the
Esc
key orCtrl-M
edit mode from command mode, hit
Enter
or click in a cell
The modal behavior of the Jupyter notebook is very efficient when you get used to it.
2.7.2.3. Inserting Unicode (e.g., Greek Letters)#
Python supports unicode, allowing the use of characters such as \(\alpha\) and \(\beta\) as names in your code.
In a code cell, try typing \alpha
and then hitting the
tab
key on your keyboard.
2.7.2.4. A Test Program#
Let’s run a test program.
Here’s an arbitrary program we can use: https://matplotlib.org/stable/gallery/pie_and_polar_charts/polar_bar.html.
On that page, you’ll see the following code
import matplotlib.pyplot as plt
import numpy as np
# Fixing random state for reproducibility
np.random.seed(19680801)
# Compute pie slices
N = 20
theta = np.linspace(0.0, 2 * np.pi, N, endpoint=False)
radii = 10 * np.random.rand(N)
width = np.pi / 4 * np.random.rand(N)
colors = plt.cm.viridis(radii / 10.)
ax = plt.subplot(projection='polar')
ax.bar(theta, radii, width=width, bottom=0.0, color=colors, alpha=0.5)
plt.show()
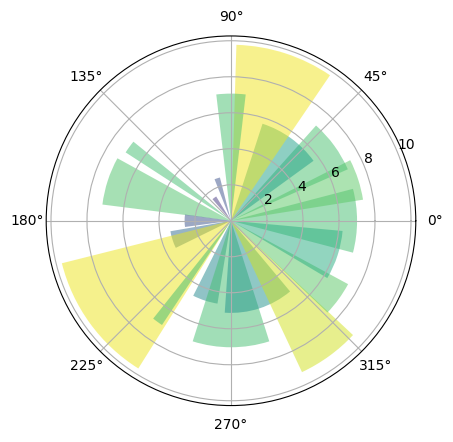
Don’t worry about the details for now — let’s just run it and see what happens.
The easiest way to run this code is to copy and paste it into a cell in the notebook.
Hopefully you will get a similar plot.
2.7.3. Working with the Notebook#
Here are a few more tips on working with Jupyter notebooks.
2.7.3.1. Tab Completion#
In the previous program, we executed the line import numpy as np
NumPy is a numerical library we’ll work with in depth.
After this import command, functions in NumPy can be accessed with
np.function_name
type syntax.
For example, try
np.random.randn(3)
.
We can explore these attributes of np
using the Tab
key.
For example, here we type np.ran
and hit Tab
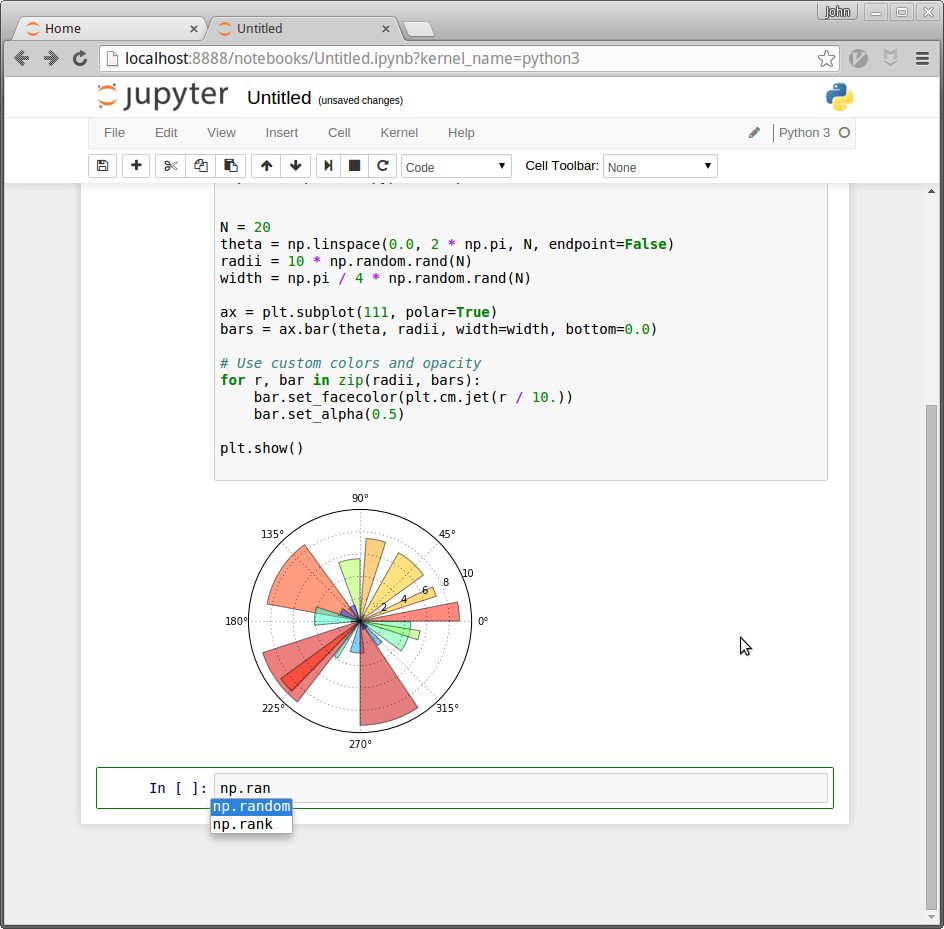
Jupyter offers up the two possible completions, random
and rank
.
In this way, the Tab key helps remind you of what’s available and also saves you typing.
2.7.3.2. On-Line Help#
To get help on np.rank
, say, we can execute np.rank?
.
Documentation appears in a split window of the browser, like so
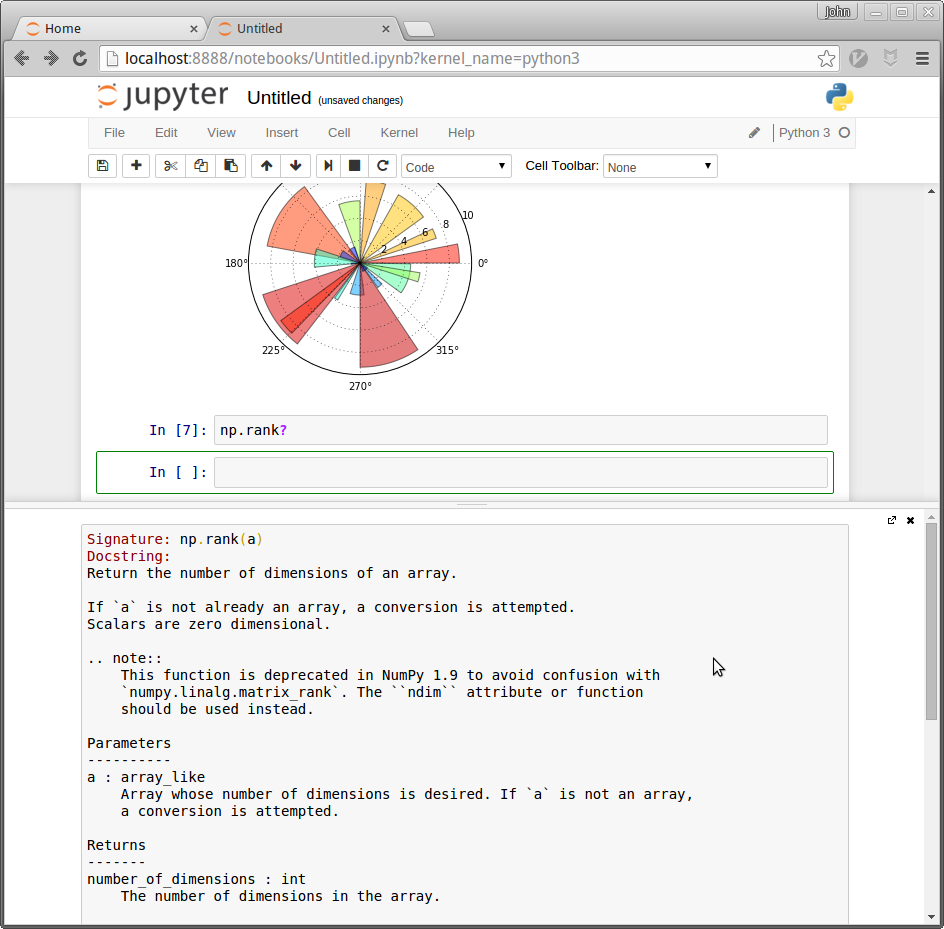
Clicking on the top right of the lower split closes the on-line help.
2.7.3.3. Other Content#
In addition to executing code, the Jupyter notebook allows you to embed text, equations, figures and even videos in the page.
For example, here we enter a mixture of plain text and LaTeX instead of code
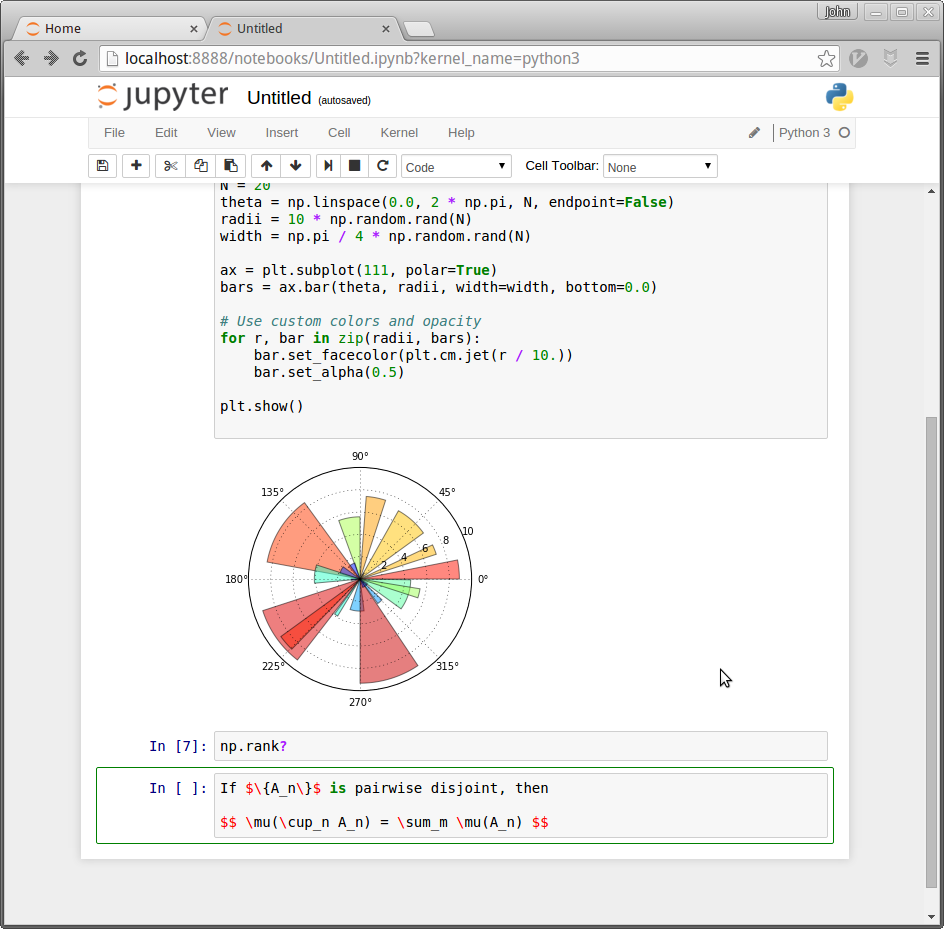
Next we Esc
to enter command mode and then type m
to indicate that
we are writing Markdown,
a mark-up language similar to (but simpler than) LaTeX.
(You can also use your mouse to select Markdown
from the Code
drop-down box just below the list of menu items)
Now we Shift+Enter
to produce this
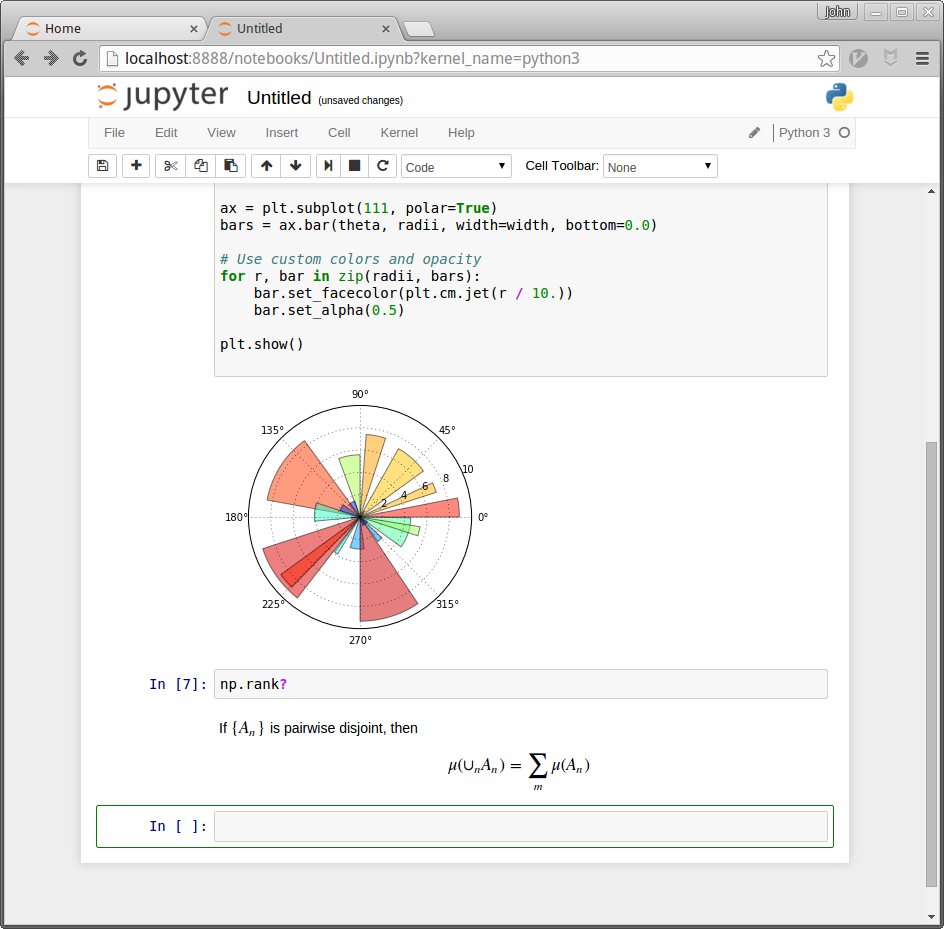
2.8. Working with Python Files#
So far we’ve focused on executing Python code entered into a Jupyter notebook cell.
Traditionally most Python code has been run in a different way.
Code is first saved in a text file on a local machine
By convention, these text files have a .py
extension.
We can create an example of such a file as follows:
%%file foo.py
print("foobar")
Overwriting foo.py
This writes the line print("foobar")
into a file called foo.py
in
the local directory.
Here %%file
is an example of a cell
magic.
2.8.1. Editing and Execution#
If you come across code saved in a *.py
file, you’ll need to consider
the following questions:
how should you execute it?
How should you modify or edit it?
2.8.1.1. Option 1: JupyterLab#
JupyterLab is an integrated development environment built on top of Jupyter notebooks.
With JupyterLab you can edit and run *.py
files as well as Jupyter
notebooks.
To start JupyterLab, search for it in the applications menu or type
jupyter-lab
in a terminal.
Now you should be able to open, edit and run the file foo.py
created
above by opening it in JupyterLab.
Read the docs or search with an internet search engine to find more information.
2.8.1.2. Option 2: Using a Text Editor#
One can also edit files using a text editor and then run them from within Jupyter notebooks.
A text editor is an application that is specifically designed to work with text files — such as Python programs.
Nothing beats the power and efficiency of a good text editor for working with program text.
A good text editor will provide
efficient text editing commands (e.g., copy, paste, search and replace)
syntax highlighting, etc.
2.9. Visual Studio Code#
Visual Studio Code is an open-source (and free) IDE created by Microsoft. It finds great use in Python development.
VS Code is an extremely popular text editor for coding and easy to use out of the box and has many high quality extensions.
The most notable features of Visual Studio Code include:
One of the best smart code completion is based on various factors
Git integration
Code debugging within the editor
It provides an extension to add additional features like code linting, themes, and other services
2.10. Python in the Cloud#
The easiest way to get started coding in Python is by running it in the cloud.
(That is, by using a remote server that already has Python installed.)
One option that’s both free and reliable is Google Colab.
Colab also has the advantage of providing GPUs, which we will make use of in more advanced lectures.
Tutorials on how to get started with Google Colab can be found by web and video searches.
Most of our lectures include a “Launch notebook” button (with a play icon) on the top right connects you to an executable version on Colab.
2.11. Scripts vs. modules#
One concept related to script mode we want to clarify is the distinction between Python “scripts” and Python “modules”.
In short, any Python file (i.e., a file with the extension .py
) can be either a script or a module, and whether
it is one or the other depends on how you use the file. In short, scripts are Python files that contain code that
actually does something (e.g., compute the average of a series of numbers, clean up a messy file, etc.) while modules
only contain code that define things.
For example a simple module (example_module.py
) is shown below. If you’d run the module, nothing will happen.
# An example module with a single function
def average(arg1, arg2):
""" Computes the average of two numbers. """
result = (arg1 + arg2) / 2
return result
A very simple script (example_script.py
) that use the module (example_module.py
) and is in the same directory of the module is:
from example_module import average
a = 5
b = 10
average_number = average(a, b)
print(f"The average of {a} and {b} is {average_number}")
The script computes the average of two (predefined) numbers (i.e., it does something). The module file only defines a function
(average
). Indeed, if you’d run the script, several things will be done (i.e., the average of a
and b
will be computed and
the result will be printed).